"Only when the tide goes out do you discover who's been swimming naked."
- Warren Buffet
This quarter, I found myself struggling with inspiration on what to cover. Q3 was busy in many ways, particularly with significant developments on the AI front:
No peak here yet!
But outside of AI, venture-wise, it wasn't a blockbuster quarter with most of Q3 coming over the summer season. Deal volume seems slightly down (~10%).
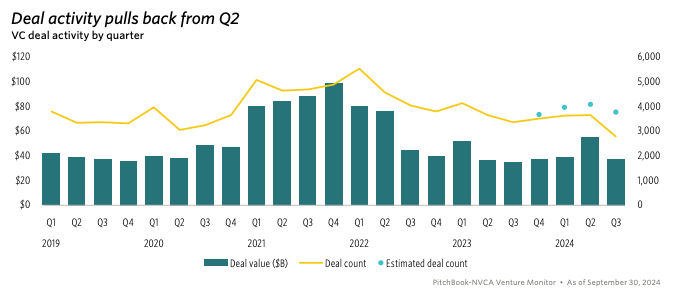
In a shorter than normal update I thought I'd put some quick thoughts to paper ahead of the larger Q4 and prospective 2025 update early-Jan. It's going to be a banger.
*Election update: I aim to keep political commentary in these updates minimal, especially as many policy details are yet to be defined. Overall, I believe Trump’s election could be positive for venture capital and the tech sector, given the likely emphasis on deregulation and a more favorable environment for mergers and acquisitions—both of which will benefit tech. I’m considering buying small-cap stocks (tech and otherwise) to take advantage of this potential shift, in addition to my WCLD 0.00%↑ long. I also bought XBI 0.00%↑.
Market Update - Number of Investors & Exits Falling
In my second update of 2023, I discussed the apparent oversupply of venture capitalists in the market. I’m cautiously optimistic to report new data suggesting that we may be nearing a correction on the staffing front. Anecdotally, we’ve seen significant shakeups, such as Index Ventures defenestrating 5 of their 9 SF based partners -- a surprising move coinciding with their $2.3 billion fundraise. This reflects the state of the market: while the number of investors may have peaked, dry-powder remains high!
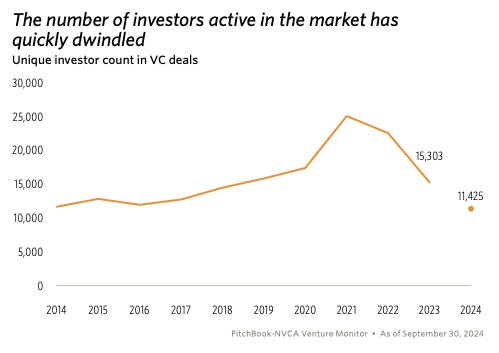
What hasn’t changed is the sheer amount of dry powder available—it remains at an all-time high. However, in my view, the set of investment opportunities has narrowed significantly since 2021 and 2022, declining by an estimated 60–70%.
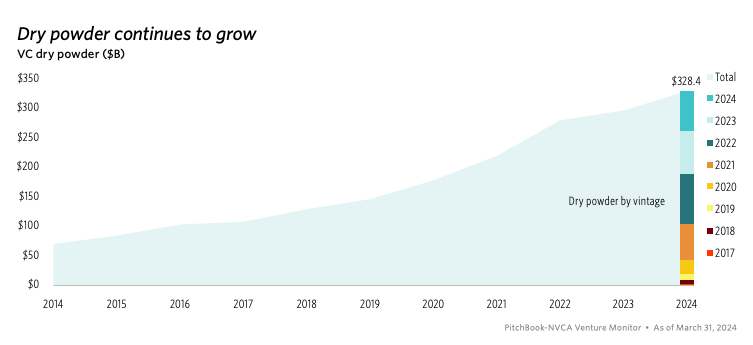
Most of the decline in the number of investors is due to a slowdown in small, first-time funds.
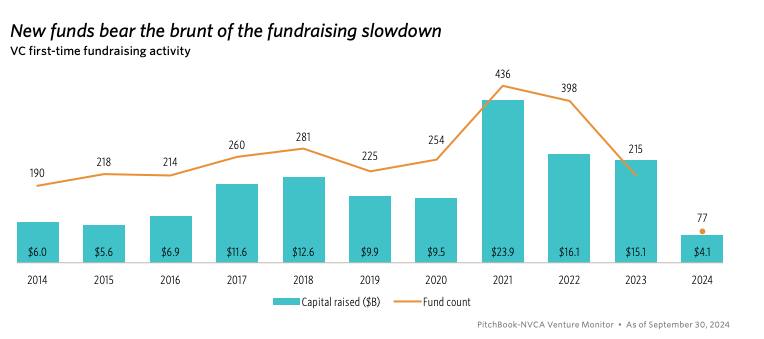
All of this unfolds amidst a lack of exit activity that has persisted over the past three years.
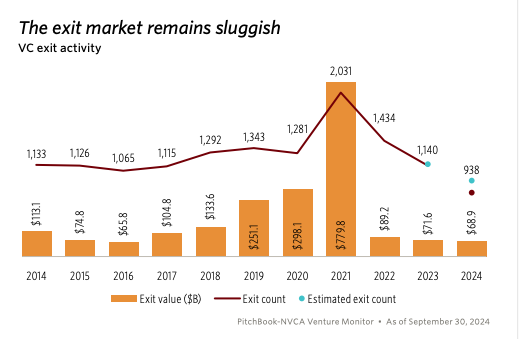
This is the core challenge: the industry faces a lack of exits while grappling with the rise of ever-larger funds at the high end of the market. Every meeting I have with LPs now highlights this contradiction in liquidity availability. If IPOs and M&A activity remain scarce, and companies continue to stay private longer, new mechanisms will be needed to provide LPs with liquidity in their venture holdings. We either need to address IPO aversion or adapt the ways VC funds achieve liquidity. Selling positions in portfolio companies on the private market will likely need to become commonplace, potentially leading to the growth of funds specializing in this area. Ironically, the fund secondary market seems to be the only private market untapped by VC mega-funds, which remain focused on traditional lines of business.
It’s remarkable to reflect on the unique moment we’re experiencing in the venture world. The number of individual investors is shrinking, opportunities have dwindled, exits have stalled—yet we have more dry powder than ever, increasingly concentrated among the top firms. What a world.
Venture and Dumb Money
The incongruence described above led me to reflect, during a call with an LP, on the unique challenge that exuberance in venture capital markets poses to investors—a challenge that is nearly unparalleled in any other asset class.
I’ve previously shared a piece of wisdom from an early mentor about capital excess: “The remedy to an excess capital environment is that capital gets destroyed” (h/t Patrick Kenary). In most other financial markets, this excess capital—often allocated by what might be called "dumb money"—can be exploited by others. In public markets, you can short stocks; in private equity, you can flip or recapitalize companies; and in real estate, you can sell properties, short debt, or short CDOs, among other strategies.
In venture capital, however, the asset class offers few avenues to profit from the presence of “dumb money.” Secondaries are difficult to execute, there’s no way to short stocks, and no listed debt exists. Instead, dumb money often becomes the top bidder, setting the price for all prospective investors. While an excess of dumb money in most other asset classes creates opportunities, in venture, it acts as a headwind. I used to refer to these players as “venture terrorists” because they blow up returns for everyone. In the rush to deploy capital, I see this dynamic playing out in many AI markets today.
At 8VC, our remedy has been to return to our roots: investing very early, before product-market fit (PMF), to capitalize on the lower cost of capital our companies enjoy when they reach their inflection points. This is the only strategy we’ve identified that indirectly benefits from the presence of “dumb money.”
OpenAI COGS and the Economics of LLMs
The Information recently published a fascinating article detailing OpenAI's financials, offering insight into the company I've never seen before. It provides a clear look into the finances of the leading AI model provider in today's market.
Since it’s paywalled I won’t share the data here, but a quick Google Image search will bring it up if you’re curious.
What immediately stands out is the staggering loss for a business operating at such a large scale. While substantial R&D and CAPEX investments are happening internally, they don’t tell the whole story. Many of you might recall my earlier point that these models are likely among the most quickly depreciating assets ever, with each remaining at the frontier for only a few months. This reality significantly challenges the unit economics of the business.
With this data, I analyzed what the margins for such a business might reasonably look like. Based on a few assumptions—particularly, that the useful life of a trained model is about 6 months (with training costs fully depreciated in COGS), that 70% of training compute is allocated to production and 30% to R&D (excluded from gross margin), and that data costs are split 50% as one-time expenses (depreciated over 5 years) and 50% as licensing fees — I arrived at a fairly shocking -38% gross margin. Even when considering only the obvious COGS components, such as compute costs for running models, the Microsoft revenue share, and hosting expenses, the gross margin still comes out to just 22%. So, I don’t think I’m too far off in my estimation!
If we evaluate the business in terms of contribution margin—and generously assume that costs like compute for training models are entirely fixed—the contribution margin comes out to 22.5%. This means that, assuming headcount remains unchanged, the company would need to generate an additional $20 billion in revenue just to break even. That’s an astonishing scale!
I suspect my contribution margin estimate might be too high, or the company may plan to expand additional money-burning business lines. Reportedly, losses are expected to increase as revenue scales over the next few years. While the company does incredible work, it is undeniably very costly to operate.
This doesn't look like a software company at the moment!
Model CAPEX with Catastrophic Forgetting
One argument for improving OpenAI’s profitability over time is that, as models stabilize, the yearly compute expenditures required to train and maintain them—currently around 50-75% of revenue—should decrease. This would mean the models could achieve a longer useful life than they currently have, which is just a few months. The company could then transition from an R&D-heavy mode to a more maintenance-focused mode, creating an opportunity to start harvesting profits.
I don’t see many people discussing this, but my intuition is that there are two major hurdles to predicting sustainably lower compute costs—even if model architectures stabilize. These hurdles revolve around keeping knowledge cutoff dates recent and integrating new data. If you look at the LMSYS leaderboards, most models are not up to date, with cutoff dates ranging from late 2023 to early 2024.
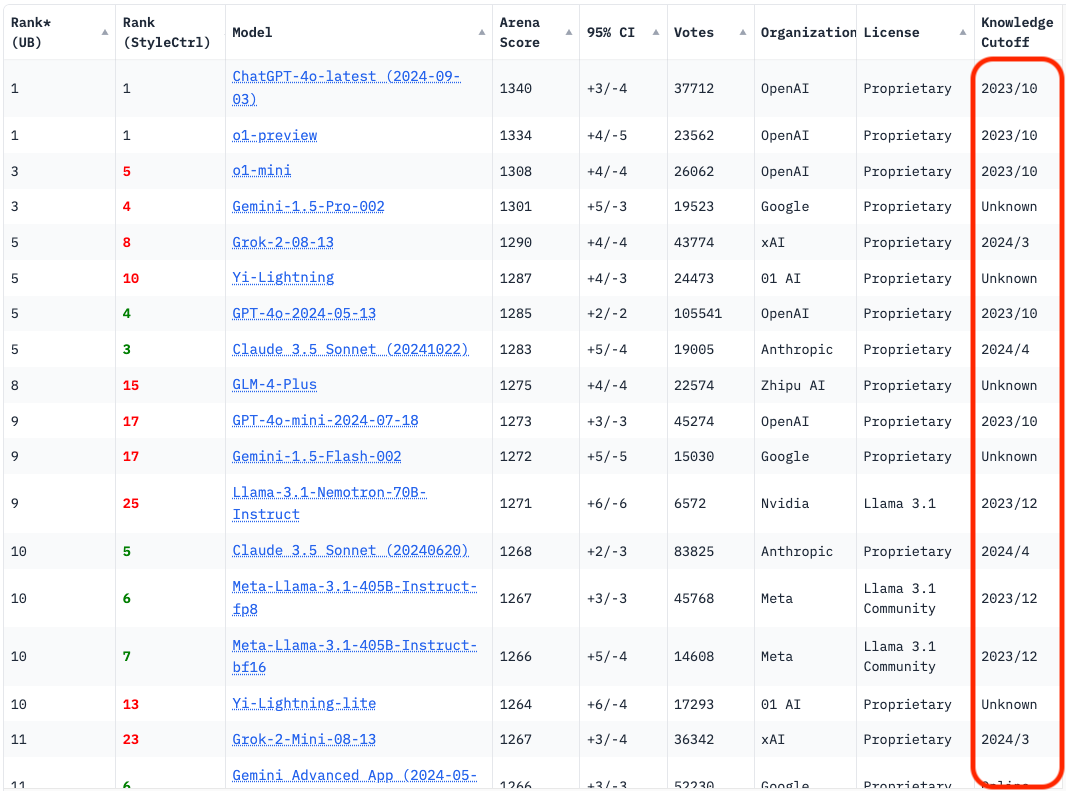
This is curious, isn’t it? If keeping knowledge cutoff dates recent were straightforward, most providers would certainly do it. I believe the challenges here stem from issues like catastrophic forgetting and post-training constraints, along with data quality and curation—though the latter is a separate concern.
I don’t hear many researchers discussing catastrophic forgetting, so I suspect it’s either a significant problem or not an issue at all. To understand the concept, consider that during pretraining, the training data order is randomized to reduce instabilities. However, when models are fine-tuned on a subset of data or continuously pretrained (CPT) on subsets, catastrophic forgetting can occur. This happens when the model overfits to the new data and loses knowledge from its original pretraining corpus. I believe this is one reason why knowledge cutoff dates aren’t regularly updated—CPT on just a subset of the training data can degrade overall performance. As a result, full, costly pretraining runs might be required to update the model’s cutoff. While there are techniques to mitigate this issue, I’m uncertain how effective they are at the frontier level.
The second issue I’ve heard is the difficulty of adding new knowledge to a model that has already undergone post-training. Academic attempts to "unlearn" certain post-training steps have largely been unsuccessful. As a result, updating the knowledge cutoff would likely require updating the base model itself, which in turn necessitates redoing the alignment process from scratch. Post-training reportedly involves numerous human-driven steps, making it both time-intensive and expensive.
The TL;DR of the above (and I’m about 80% confident in this, as much of the knowledge on training and alignment is proprietary—I’m piecing this together from expert conversations and inferences) is that keeping knowledge cutoff dates semi-recent will likely require large, ongoing training and alignment runs, even if model architectures, sizes, and datasets stabilize.
If no effective techniques are developed to address these challenges, the compute expenditure for foundation model companies is likely to remain persistently high. Models will continue to depreciate quickly, as updating knowledge cutoff dates periodically to stay current will remain necessary. A tough business indeed!
GPU Price Crash
Eugene Cheah, an entrepreneur known for his work on RWKV, wrote an insightful piece about the H100 (NVIDIA's flagship AI GPU) rental market. He explains that outside the tier-1 cloud providers, H100 rental prices have dropped significantly—from $4.70–$8 per hour in early 2023 to just $1–$2 per hour today, a decline of over 50%. Many tier-1 providers, such as Amazon and CoreWeave, still charge $4–$5 per hour, but these rates don’t reflect the broader market. This represents a stark shift from 2023, when investors were scrambling to secure compute time for deals!
This is particularly noteworthy because it renders the economics of buying and renting GPUs quite unfavorable. With rental prices dropping to $2–$2.85 per hour, the resulting internal rates of return (IRRs) are very low—likely too low to be financeable.
This is an important trend to monitor, as unfinanceable IRRs could lead to declining GPU demand for NVIDIA. NVDA 0.00%↑ has been the biggest beneficiary of the AI wave so far, and as I mentioned in my last update, I believe we’re nearing a peak. I don’t think we’re there yet—there’s still significant optimism surrounding their next generation of Blackwell chips, and I’m aware of a few more megaclusters under construction. However, GPU rental rates could serve as an early warning sign, indicating potential weakness in production AI demand relative to CAPEX supply.
As a reminder, I’m extremely bullish on AI over the next 10 years, but I remain skeptical that near-term developments will meet the current inflated expectations. That said, I will let data guide my perspective. I’ll continue to monitor how AI demand in production evolves and track indicators like NVDA 0.00%↑ and GPU rental rates to quantitatively assess demand.
ASIDE: I need a good time series and datasource for GPU rental costs if anyone can provide it
Conclusion
I plan to write a more comprehensive update in my end-of-year note and 2025 lookahead, where I’ll address a few topics I’ve yet to cover—such as my perspective on different types of market leadership and how startups should evaluate their natural growth rate. Until then, onwards!
Interesting Things to Read/Watch:
Ilya Sutskever recently told Reuters results from scaling pretraining have plateaued. Regular readers heard it here first!
Great read. Particularly love the point about the ‘short dumb money’ trade being to invest earlier and take advantage of the distorted cost of capital that comes in at later stages. Very cool
Fantastic analysis as always. What would the VC market charts look like without AI deals? Now is a great time to find hidden gems in less buzzy sectors that aren't receiving as much attention from dumb money.